
Hybrid NVH Modeling
The ever-increasing demand for shorter development times and cost savings means that important design decisions must be made early in the development process, promoting the use of virtual prototypes. Combining simulation and testing can further facilitate the idea of saving time and reducing costs. Also, the accuracy of the results can increase compared to approaches solely based on numerical models on the one hand and experimental results on the other. It is vital to choose the optimal approach depending on the task and data availability (to build virtual prototypes), methods (to model certain physical phenomena), and physical prototypes. In a connected world, where the development process is distributed across different companies, the availability of data and physical prototypes becomes even more critical: Companies need to share product features to create system models but may not want to share development knowledge or product design details.
A real-life example
In this blog post, using an e-bike as an example, we show you how a combined method of measurement and numerical simulation to predict and analyze the Noise, Vibration, and Harshness (NVH) properties caters to all the needs and constraints mentioned before.
In this specific case, we virtually install the electric drive unit of an e-bike already in series production into a numerical model of a new e-bike prototype, as shown in the image below.
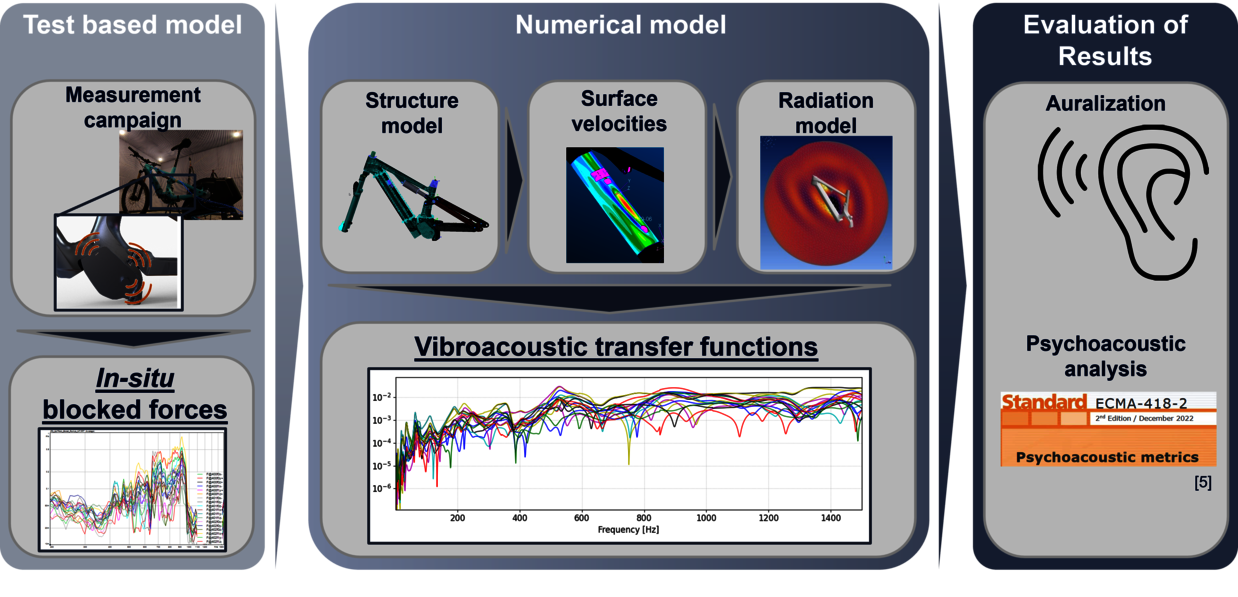
Fig. 1: The structure of the hybrid model in our example
The test-based model
The electric drive unit’s excitation and structural dynamics are derived from experiments using the Equivalent Forces (EFs) method, better known as in-situ blocked forces [1, 3]. You might know this kind of source description from experimental transfer path analysis. To transfer such “source descriptive forces” from a test case to a simulation model, these forces must exclusively be a property of the source - our drive unit - rather than belong to the receiving structure – the assembly that holds the drive unit.
That means the in-situ blocked forces of the electric drive unit are the same for the electric drive mounted in a prototype or a series-production e-bike – and that’s why we need in-situ blocked forces instead of directly measured operational forces, which are property of both the source and the structure that holds the source.
The numerical model
Additionally, we build a numerical model to predict the structural dynamics of the e-bike prototype using a bottom-up method. In this case, the term bottom-up method means that the system is divided into several sub-models that we can validate separately. For every sub-model, the level of detail and the degree of abstraction match the available data and the problem we want to solve. The numerical model consists of the drive unit, battery, frame, fork, rear swingarm, front wheel, and front and rear shock absorber. In this case, we assume that the e-bike frame, especially its lower tube, radiates the most acoustic energy, so it needs to be modeled as a flexible part.
One challenge was the frame made from carbon-fiber-reinforced plastic, which meant we had to consider the different layers' thickness, orientation, and material properties. We assumed that the battery and the drive unit also affect the e-bike’s structural dynamics, but there is no data available for these components. Thus, we performed modal analyses as preliminary studies to determine how the respective components’ structures behave.
The hybrid model
We coupled the structural dynamics’ numerical model with a model that predicts the radiation by means of the surface velocities on the frame (see fig. 1). Both models add up to a set of vibroacoustic transfer functions from different excitation points at the interface between the electrical drive and the frame to different microphones around the e-bike. The combination of the vibroacoustic transfer functions predicted by numerical simulation and the in-situ blocked forces derived from actual measurements let us predict the real sound of the e-bike prototype.
In the freely accessible papers [1, 2], we validated the predictions of our hybrid NVH modeling approach through measurements, enabling us to evaluate the accuracy of the achievable predictions (see fig. 2).
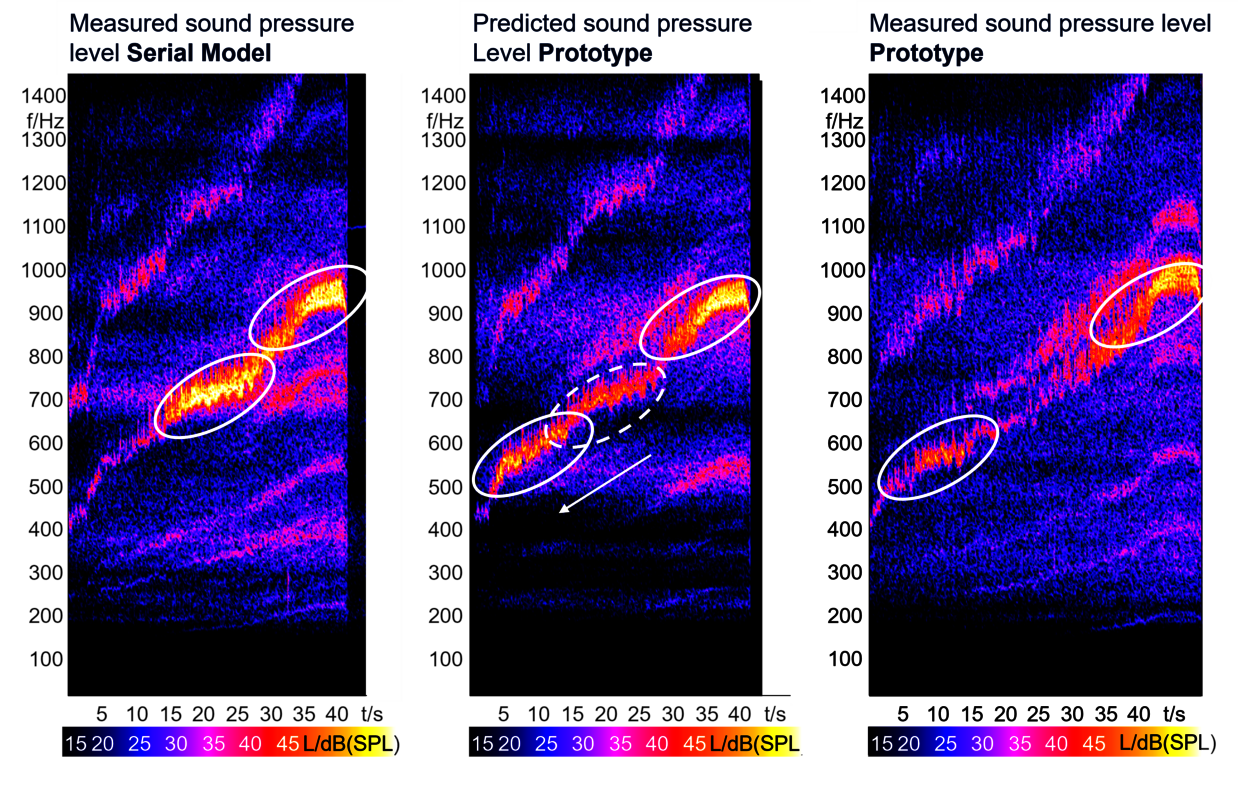
Fig. 2: Comparison of measured and simulated sound pressure levels (a. measured serial model, b. calculated prototype, c. measured prototype).
In the measurements of the serial model, we can identify two potentially critical operational states, marked in the left of fig. 2 with white ellipses.
The first mode of operation is in the middle of the run-up, and the second at the end. Combining the numerical prototype model with the experimentally derived excitation of the serial drive unit, we can predict that the first potentially critical mode of operation is shifted from the middle of the run-up to the start of the run-up (see fig. 2 in the middle). The validation measurement of the prototype (see fig. 2 on the right) shows that we were right.
Perception and psychoacoustics
How humans perceive sound is often more important than the overall sound pressure level. Human perception is highly individual and is linked to how well the user’s expectations of the sound and the actual perceived sound match. Therefore, generalization of how “good” a sound is can be difficult. However, psychoacoustic parameters developed and validated by jury tests are an established tool in experimental measurements to quantify the human perception of specific acoustic phenomena like tonality, roughness, and loudness.
Using simulation, we can evaluate how humans perceive a product’s acoustical properties long before it exists in the real world. However, high accuracy is mandatory to make analyzing numerical results with psychoacoustic parameters possible and meaningful. Fortunately, numerical computations continuously become better because
- hardware performance and availability increase, allowing for more detailed models,
- more sophisticated methods are developed to model specific physical phenomena, and
- hybrid modeling approaches enable coupling test-based models with numerical models (like the one presented here), leading to more flexibility in selecting the optimum method/model for the specific task or sub-task.
In fig. 3, you can see how psychoacoustic analysis of numerical results matches and enhances the analysis solely based on the sound pressure level discussed in the previous chapter.
The analysis of perceived tonality shown in fig. 3 corresponds to the potentially critical modes of operation identified during the analysis of sound pressure level shown in fig. 2. The measurement of the serial model depicted in black shows a peak in the middle of the run-up (80-85 rpm) and rises to a maximal value towards the end of the run-up. The hybrid model (red curve) predicts that the peak in the middle of the run-up is shifted to lower speeds for the prototype. The measurement of the prototype confirms this prediction (green curve).
Sound of the measured serial model
Sound of the simulated prototype
Sound of the measured prototype
This is only a single example of the results we provide in our papers [1,2], but it already demonstrates how you can take a huge step from pure imagination of your future product’s acoustic properties to the analysis of the predicted human perception using our hybrid NVH modeling approach and psychoacoustic analysis.
You can even listen to the results and experience your product before it exists as a prototype, giving you a strong competitive edge.
Sources
[1] M. Wegerhoff, T. Kamper, H. Brücher, and R. Sottek, “Hybrid NVH modeling approach: How numerical and experimental methods complement each other”, Engineering Modelling, Analysis and Simulation, vol. 1, Jan. 2024. https://doi.org/10.59972/4f576ll9
[2] T. Kamper, M. Wegerhoff, H. Brücher and R. Sottek, “Hybrid NVH modeling approach: High quality of NVH results enables psychoacoustic analysis of numerical computations,” Engineering Modelling, Analysis and Simulation, vol. 1, Jan. 2024. https://doi.org/10.59972/pvt6hrtg
[3] M. V. van der Seijs, D. de Klerk, and D. J. Rixen, “General framework for transfer path analysis: History, theory and classification of techniques,” Mechanical Systems and Signal Processing, vol. 68–69, pp. 217–244, Feb. 2016, doi: doi.org/10.1016/j.ymssp.2015.08.004.